Research
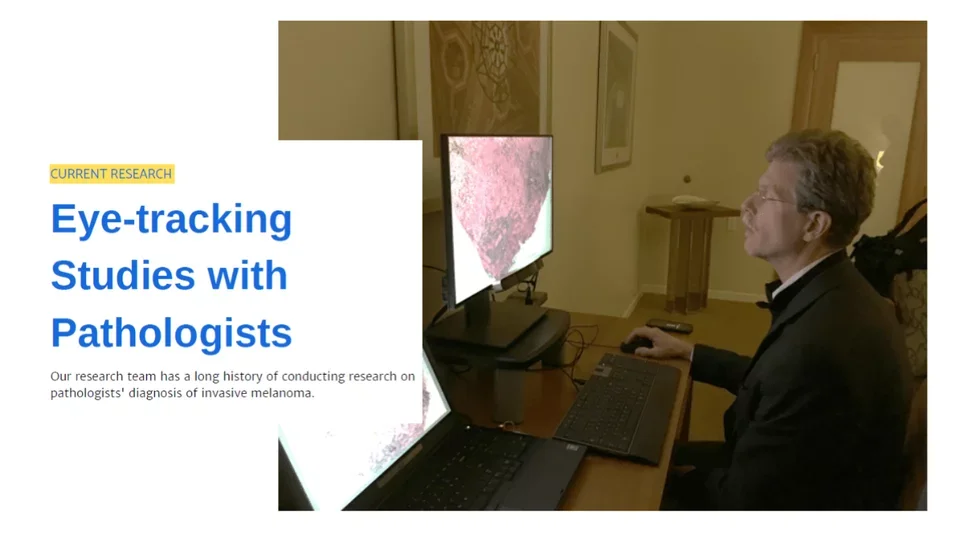
Above is a photograph of Dr. Don Weaver, Co-Principal Investigator on multiple grants, taken from the RAPID-PC study using our contact-free eye-tracker.
Featured Current Project | Reader Accuracy in Pathology Interpretation and Diagnosis: Perception and Cognition (RAPID-PC)
Accurate pathologist diagnoses are the cornerstone of both patient care and cancer research. The diagnostic process requires complex visual perceptual tasks interacting with cognitive processes, yet little research has been devoted to understand and potentially improve how skills needed for accurate diagnoses are acquired.
In The Invisible Gorilla, Drs. Chabris and Simons explain how our brains trick us into thinking we see and know far more than we actually do. In their experiment, they find that when we are paying very close attention to one thing, we often fail to notice other things in our field of vision, even those which are the most obvious.
A new study led by Dr. Joann Elmore, "Reader Accuracy in Pathology Interpretation and Diagnosis: Perception and Cognition (RAPID-PC)", explores this process in cancer research by evaluating the underlying processes of perception and cognition in the interpretation of breast biopsy images.
While millions of breast biopsies are obtained each year, prior research by Dr. Elmore and colleagues identified a concerning level of diagnostic disagreement and errors in the interpretation of breast biopsies related to cancer screening.
Under a five-year grant from the NIH's National Cancer Institute, the RAPID-PC study will contribute to understanding how diagnostic pathology expertise develops over time and will study the types of diagnostic errors made by both residents in training and experienced pathologists while interpreting breast biopsy images.
Breast Cancer Research
The recent study "Assessment of Machine Learning of Breast Pathology Structures in for Automated Differentiation of Breast Cancer and High-Risk Proliferative Lesions" demonstrates the importance of adopting digital whole slide imaging in clinical settings to improve diagnostic accuracy, especially under the context of analyzing breast biopsy specimens. To do this, the study developed and evaluated computer vision methods to assist pathologists in diagnosing the full spectrum of breast biopsy samples, from benign to invasive cancer.
An additional research study includes:
AI for Improved Breast Cancer Screening Accuracy: External Validation, Refinement, and Clinical Translation (R01 CA240403): In this project, we propose a unique academic-industry partnership to validate, refine, and translate a highly accurate artificial intelligence-based algorithm that resulted from the Dialogue for Reverse Engineering and Assessments and Methods (DREAM) Digital Mammography Challenge.
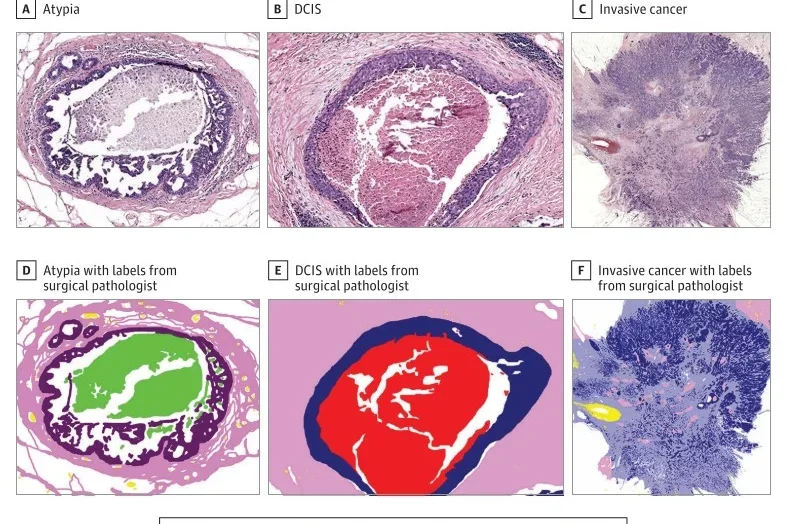
Pictured: A collection of images demonstrate the classification process using machine learning techniques. Colors communicate background, normal stroma, malignant epithelium, blood, benign epithelium, secretion, desmoplastic stroma, and necrosis.
Melanoma Research
Reducing Errors in the Diagnosis of Melanoma and Melanocytic Lesion (REMI) (R01 CA201376): This study evaluates diagnostic accuracy to identify sources of diagnostic errors and strategies to reduce errors in skin biopsy interpretations.
Machine Learning
Improving Melanoma Pathology Accuracy Through Computer Vision Techniques (IMPACT) (R01 CA200690): This study uses computer machine learning techniques to improve our understanding of causes of diagnostic errors during interpretation of skin biopsies.
A Unified Machine Learning Package For Cancer Diagnosis (U01CA231782): This study develops a unified software package for sharing image analysis and machine learning tools to improve the accuracy and efficiency of cancer diagnosis.
Applying AI to Improve Melanoma Diagnosis (Melanoma Research Alliance) (622600): This study applies novel computer machine learning methods to detect histologic and morphometric attributes of melanocytic neoplasms.
INSPIRE Registry (COVID-19 Research)
The Innovative Support for Patients with SARS-CoV-2 Infections (INSPIRE) Registry is a multi-institution collaboration that looks at the long-term health outcomes of patients under investigation for SARS-CoV2. The study uses a digital platform (Hugo Health) to longitudinally track patients' self-report data as well as the process and outcome of care in their electronic medical record (EMR). For more information about the study, visit INSPIRE COVID-19 Study.